Just this morning, at a roundtable of IR professionals from well-known private equity and venture capital firms, I asked the question “how many of you are using investor relations software to score or predict the likelihood of prospects to commit to funds you’re raising?”
For a good ten seconds, it seemed as if there would be no reply whatsoever from the participants, but ultimately one brave soul did offer up something more or less equivalent to a manually calculated, somewhat arbitrary, and highly subject to interpretation method they use to figure how engaged a prospect is or how likely it is for a prospect to actually convert via commitment.
I left the early part of my career in venture capital not to shame how firms in this market behave, but rather to take advantage of an amazing opportunity to provide technology to these firms; the result of which would create opportunities for the firms themselves to use technology as a strategic weapon. It is my belief that there have never been greater opportunities for investor relations software to change the way PE/VC markets operate than there are today, and I want to start with this concept of predictive scoring, just one basic example of the application of technology.
Measuring how engaged a fundraising prospect is with any given GP raising capital is something that is proprietary; in a world where enrichment providers abound and help save us the mundane, manual entry of data that is objective, there’s simply no way for measuring engagement that doesn’t involve proprietary technology. Said another way: an enrichment provider like Pitchbook, Datafox, Preqin, or CapitalIQ is neither fit nor able, to tell you about the correlation between the messages and channels you use to the likelihood of closing the target. Not in terms of raising capital nor sourcing investment targets.
There are a number of firms we work with that arrive at this, however, and they do so via a modern mix of CRM (the source for proprietary actions taken and other data) and proprietary analysis that most often takes the form of modern analytics applications within the investor relations software. Where yesterday’s CRM is oftentimes seen as a management-driven hassle that users have no choice but to use, today’s CRM is an important provider of critical activity-based data and their associated outcomes, and which provides data to capable analytics applications that in turn start to help us understand patterns and correlations between activities and outcomes.
Let’s look at a basic example of this within the context of deal sourcing efforts. On the surface, every outbound firm has its deal sources, and a certain number of them will be considered “proprietary”. Many firms even have a surface-level assumption about how many of those deals — from a given source — they’re able to close. Note that I’m deliberately choosing to avoid using the word “understanding” in favor of “assumption”. The way I’m defining “understanding” in this case can be widely interpreted, and a simple number of deals closed is a perfectly fair definition by anyone’s standards, but it is my belief that there’s a definition that most firms would prefer. That definition considers the following:
- whether those deals are deals that are likely to close to begin with
- where deals from this source get stuck and/or how much time is spent to get them closed
- whether there are efforts and activities that correlate to increasing the volume of deals from the given source (if it is so desired!)
- whether the time spent on deals sourced from a given source is actually worth the all-important opportunity cost of lesser-considered sources
I’m attempting to stay relatively high-level with these considerations, but the last question above is the most important because it begins to consider whether there is a more effective use of the same resources. An easy way to think about this is to understand — through proprietary data — whether there is a “better” source for your efforts. In this case, “better” may not mean higher volume; it may mean a greater likelihood of success, or greater return on investment. More importantly, consider whether increasing the activity and engagement with that source has the effect of increasing the volume of higher quality deals.
Let’s pause there for a moment, and take the same proprietary data and analysis and port it to our understanding of fundraising efforts. Which activities lead to the highest conversion? Which attributes (location, size, mandate, etc) lead to an increased conversion with new LPs? Which messages are most effective? What is the typical engagement path and how long does it take to get to conversion? Which activities should we be taking and when?
But where proprietary technology and data begin to get extremely powerful is when we combine these two examples and begin to tell fundraising prospects a data-driven story about how our story is unique and how we’re differentiated in our ability to find the best opportunities. If that is where it begins, it is most certainly not where it ends; armed with the ability to understand our proprietary data and analysis of it, there’s no reason we can’t expect to begin to better understand how to win more competitive deals, both in terms of understanding deal dynamics and in terms of our ability to communicate what makes us differentiated when presenting to management teams.
In a time where the world is attempting to automate as much as possible, we must be careful to understand and distinguish between the time-saving automation efforts, and the invaluable proprietary tactics and data that can’t be automated. The combination of these two, and the insight therein is where true differentiation lies.
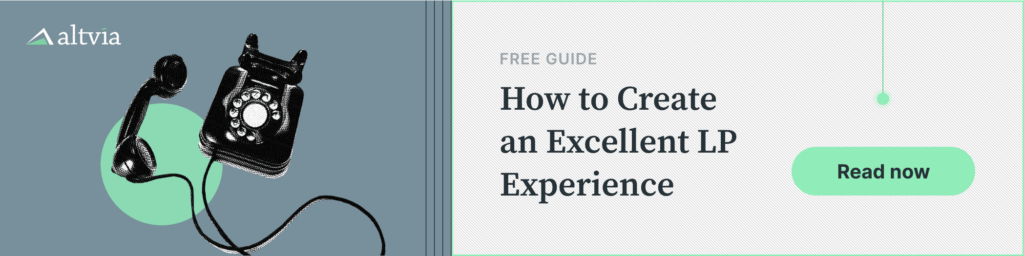