Data & Technology Guide For Private Equity Firms
A how-to guide to help you pivot from reactive responses to proactive communication.
Chapter 1: Private Equity Data & Technology Trends
Many of today’s trends in the private equity marketplace are centered around data and technology. Trending topics include how firms are able to access, use, and interact with data using more advanced technology in order to gain valuable insights about themselves, investors, prospects, potential investments, and the rest of their universe. Simultaneously, firms are tasked with navigating increased regulation and the need for greater transparency. Fortunately, technology can provide valuable operational leverage in these areas, freeing up resources so that people within the organization can focus more on strategic activities.
While Limited Partners in the asset class have become increasingly “institutionalized”, the historical range between return quartiles of recent vintage private equity funds has narrowed considerably. The combination has led to increased demands of managers from LPs, but has also led
many managers to pursue a new strategic approach to positioning and differentiating themselves, and in turn better preparing themselves to respond efficiently to these new demands.
Operational challenges differ between firms, but many of today’s most significant hurdles, according to firm executives, share a common basis in increased reporting demands: financial reporting, portfolio reporting, regulatory reporting, and investor reporting are at the top of the list. Despite firm differences, “data” is the single most pervasive challenge reported by executives at private equity firms, with nearly two out of three reporting it as their most significant challenge.
The interrelatedness of these challenges is not coincidental; the systems and processes required to facilitate stress-free reporting that meets the demands of today’s market live and die by the data upon which they’re built.
As many in the asset class wrestle with the anxiety of these challenges, Einstein’s famous words “In the middle of difficulty lies opportunity” are fueling a new evolutionary cycle for emerging and longstanding private equity managers alike. They have embraced and been energized by the realization that in order to differentiate in a quickly evolving environment, they need to be enabled to more effectively accumulate, store, and analyze data. As Altvia’s Founder & CEO, Kevin Kelly puts it, “increasingly, private equity firms are being evaluated by execution and operational excellence in addition to performance. Firms that welcome this trend will pull ahead of their peers and differentiate more successfully in the marketplace.”
What’s already becoming clear to those leading this evolution, however, is that simply throwing money at the problem can not only be expensive, but can lead to long- term commitments that, in a rapidly changing market, are just enough to take them from the front of the pack to the back of it. Instead, those that remain at the front of the pack are strategically evaluating their challenges and thoughtfully developing future-proof solutions that optimize the investment in both technology and people to develop a solution that solves for the unique challenges they face.
How Did We Get Here?
For the better part of roughly the last twenty years, the private equity industry has been active in using technology to generate data, and more recently, to consume broader data sets. However, many firms have accumulated data much less methodically than firms that are starting out today, and this reveals some important considerations:
- Firms that have been in business longer have ended up with multiple systems along the way, and there are often no integration points between those systems.
- Older systems, on their own, are oftentimes incapable of making the data contained within them relevant to data outside them. Where capable, antiquated yet costly skill sets are required to facilitate them via processes that are often manual, error-prone, and time-consuming.
While it’s reasonable to assume that large, transformational technology initiatives result in the throwing away of existing technology and starting fresh, industry leaders are actually discovering compelling reasons to consider data strategies that don’t always lead to total replacement. They’re finding success in keeping the systems that work well, finding best-of-breed replacements for those that don’t and investing in the newest innovations in technology, which focus primarily on integrating and facilitating the ability for these otherwise disparate systems to provide insights that can be turned into strategic differentiation.
In effect, the market for analytics solutions — where data and technology meet — appears to be headed for two distinct approaches to underlying systems: all in one solutions and best of breed technologies. The two are distinct, and it can be helpful to keep the differences in mind when considering a data strategy:
- An all-in-one solution provides benefits of a potentially more unified approach. There may be fewer potential complications in this approach, but it may mean settling for inferior solutions in certain areas, which may lead to risk to the strategy.
- In contrast, best-of-breed options provide the benefits of flexibility and customization with a much greater ability to adjust solutions over time. This allows the organization to align systems and technologies with changing business requirements and growth.
Considering the practical need to combine portfolio performance data, accounting data, deal flow data, and investor relations data in order to meet reporting challenges, it’s logical to expect that the benefits of an “all-in-one” or “front-to-back-office” solution will yield the greatest success. It certainly may, but this commonly-held belief is starting to give way to the realization that buyers searching for them will face tough decisions about which functional areas will have to settle for the less-than-capable pieces within the overall package. After all, whether the systems being replaced were significantly more effective — or only marginally more — than the systems being replaced, the combination of a steep learning curve together with the feeling of being marginalized may lead users to limit their use of systems, and in many cases stop using them entirely. If so, the foundational layer of a data strategy is now compromised, as the lion’s share of data that delivers insights downstream is first created by users of these systems.
A best-of-breed approach allows for the option to selectively keep, add, change, and integrate systems across vendors. Each piece of the equation can and should be considered replaceable and be evaluated against the firm’s requirements. This is especially important within the context of a broader data strategy, which may justify replacing a system that meets current needs for one that provides new capabilities that better align with the mission of the broader data strategy.
With a multitude of potential solutions in the market, it is key that each firm consider developing its own strategy, irrespective of its current systems, that focuses on problems the strategy will solve. Then those involved in the process are more effectively able to assess the available universe for solutions to those problems without compromising in key areas that all in one solutions may present.
Chapter 2: Demystifying The Process
While it’s tempting to formulate a comprehensive data strategy by skipping right to demos of the exciting new capabilities of web-based dashboard products, a lack of dashboards isn’t the problem leading data strategies to solve for. If it were, one would have to look no further than Microsoft Excel, the global leader in building charts for the last thirty-two years.
Instead, focus on what problems or gaps the strategy will address. Questions that can be helpful to this process are included in the figure to the right. →
By focusing on what you would like to be able to do as part of a data strategy, uncovering what you’re doing that’s working, and identifying issues that present gaps in getting to your end goals, you will be better equipped to find solutions to the challenges that make your operation distinct.
Having identified the specific problems you’re looking to solve, you’re one step closer to getting demos, but still not ready. Understanding what a solution to these problems includes and the basics of how it will work will be key to making the most of the time you spend getting demos.
Data strategy checklist
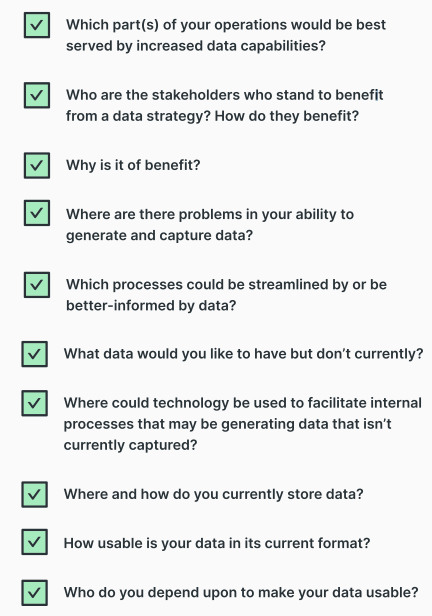
The value chain of deriving insights from data is inevitably distinct for every firm, but on a basic level, the process irrespective of the tools involved — is made up of the following steps:
1. Accessing and/or connecting to data
– How will the data be accessed?
– Manually or automatically?
– How fresh will it be over time?
2. Preparing data to be properly analyzed
– Can the data be combined with other data?
– If so, how?
– Who does this, and how error-prone will it be?
3. Performing the analysis on and/or consuming the data
– Who can do analysis?
– How do they do it, from where, and how complicated is it to do?
This may seem straightforward enough, but consider each of these steps against the problems you’ve identified and the gaps in your current situation. Consider specific examples of data-related workflows like fulfilling information requests, preparing diligence materials, reporting to partners, generating investor-facing materials, answering ad-hoc questions, etc. In considering these steps in each of these workflows, attempt to uncover the following:
- Where do we spend the most time?
- Where are we dependent upon people for specific and/ or costly skills?
- Who is involved? How much time do they spend? Is this the best use of their time?
- Where is there a risk? How error-prone is this? How do we know whether it’s right or wrong?
- Who benefits? How and why?
- What would we need to improve this? Who would need to be involved, and what do we stand to gain?
If you’re like most, you’ll discover that this simple process — even on a small scale — is littered with potential problems that you’ll want to consider in the development of your data strategy. For you to see success through this process, you must be clear on what you’re trying to accomplish, what the problems are that you need to solve for in order to accomplish it, where these problems occur, and how best to solve them.
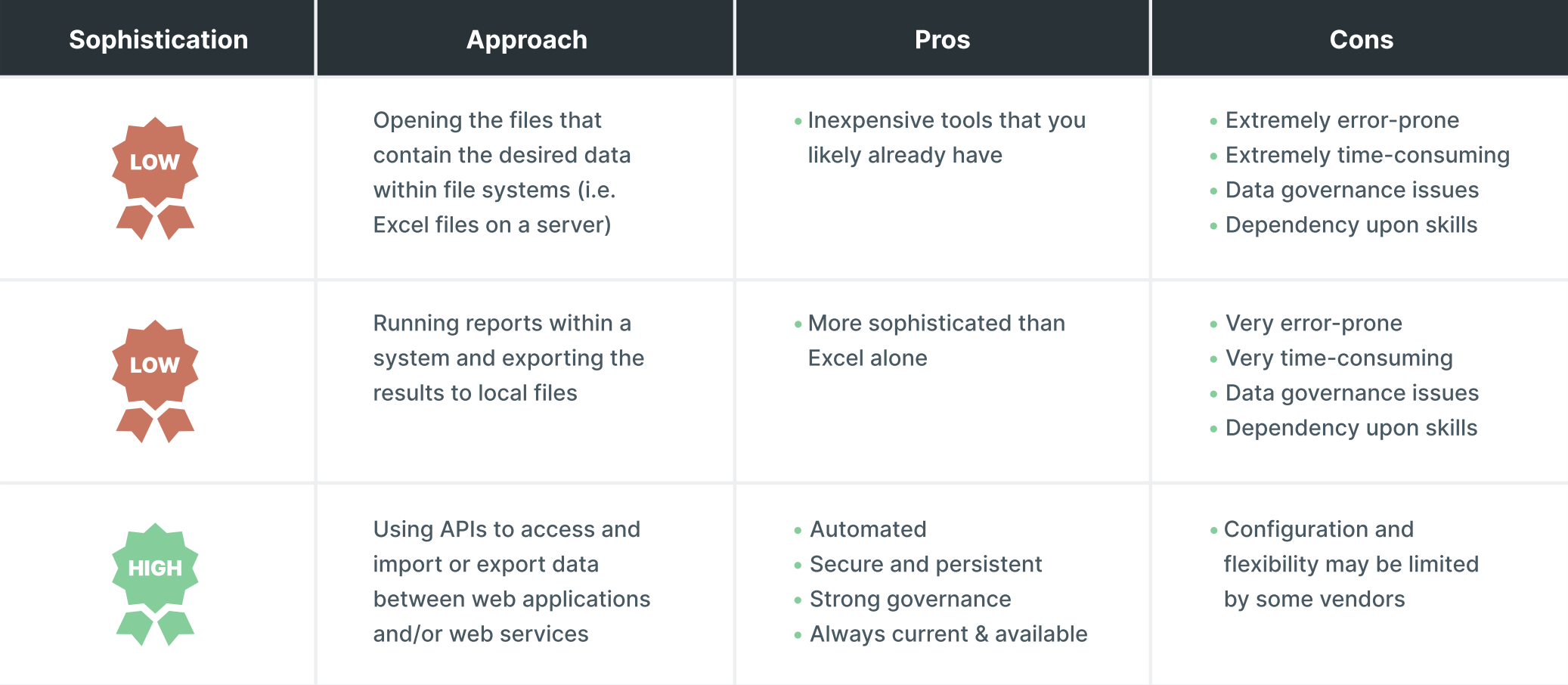
There are countless combinations of products and/or processes that this basic 3-step procedure can use to get to analytics. The formulation of a data strategy that will beat the competition must consider the problems specific to each firm, and which combination of products, services, and/or processes is best suited to solve these problems in a way that will be successful over the long-term. To that end, it’s worth being familiar with the common categories of tools and/or processes that address these steps. What follows is a high-level overview of them.
Step 1: Accessing and/or Connecting to Data
The first step in any analytics value chain is first accessing the data, and it can take many common forms. Each of these approaches has its own advantages and disadvantages in the consideration of a data strategy. While you’re likely to already have experience with at least one of these approaches, the advantages and disadvantages of each may not be obvious within the context of your strategy. Please see the figure above for a summary of them.
Key considerations for this step in the development of a solution should be framed by the time and effort required to access the data, how secure and/or error-prone it is to access it, and how repeatable and predictable accessing it will be.
Step 2: Preparing Data to be Properly Analyzed
The preparation step is arguably the most important, as proper preparation will make or break whether the analysis can be accurately and easily performed and subsequently consumed. No matter its ultimate source, all data must be prepared, and can mean doing something as simple as using Excel to correct typos or deduplicate rows.
The capabilities of modern data preparation tools, on the other hand, have evolved from Excel’s shortcomings; the limited, simpler approaches that revolve around Excel can be extremely time-consuming and extremely prone for human error. They aren’t predictable nor repeatable, and can often lead to analytical errors that aren’t always apparent in consumption.
Modern data solutions prepare data in ways that are far more sophisticated, using powerful data warehouses to store real-time snapshots of disparate data sets in one single source-of-truth location, perform persistent transformations of the data sets such that they match structure and syntax, and automatically deduplicate and prepare data on the fly for analysis and consumption downstream. Once configured, this approach persists and perpetually provides analysis that eliminates human intervention and the risks that come with error-prone processes and tools with limited capabilities.
The preparation layer is likely to be one, in identifying problems with your data, that uncovers a centralized dependency on specific skills and/or long periods of time required to meet reporting demands. In assessing their current situation against this step, many firms become aware of an unhealthy dependency upon analysts with impressive Excel skills. Then, upon seeing modern capabilities, discover that the time required of analysts to perform these analyses — together with the risky error-prone nature of them doing so — is a compelling reason to look at turning to technology to improve this step. In doing so, analysts become valuable resources that can be redeployed to other more valuable activities — in many cases ones nobody had been doing — that allow firms to become strategic in differentiating themselves.
Step 3: Performing the Analysis on and/or Consuming the Data
Properly prepared, data is now ready for its ultimate purpose — to be analyzed and consumed. This is the area that modern technology has impacted most significantly in recent years, having dramatically changed the ways in which data can be analyzed and consumed, the scale at which data can be interacted with, and even the influence of Artificial Intelligence technology to alert and predict insights based upon data without humans even having to ask the questions.
One of the biggest modern innovations in this regard comes from tools that provide business users, who don’t possess technical data skills, to intuitively complete complex analyses without the help of IT, and to do it in real time. While the business intelligence and analytics category has traditionally been IT-led and required complex resources like data analysts and data scientists, the entirety of the data analytics market has now shifted to empower business users, without the dependencies of IT skills, to create and consume their own analyses. This “self-service” model has led to dramatic improvements in the time it takes to get answers from data, to discover insights in an engaging, interactive way, and in turn has dramatically reduced the bottlenecks associated with de- pendencies on people, skills, and process to get there.
Gartner’s Magic Quadrant for Business Intelligence and Analytics Platforms, issued in February of 2017, shed light on this shift, which it had been tracking in previous years’ versions of the same report. The opening commentary reads “The business intelligence and analytics platform market’s shift from IT-led reporting to modern business-led analytics is now mainstream.” Now mainstream, industry-leading private equity firms are em- powering their business users to discover insight in their data themselves, in record time, and in ways that seamlessly combine data across the entire organization. In so doing, the data and reporting challenges they once faced are now forgotten, having instead turned their attention to how to take their newly-enabled capabilities on the offensive to beat their competition and to provide a new level of differentiated service to their partners.
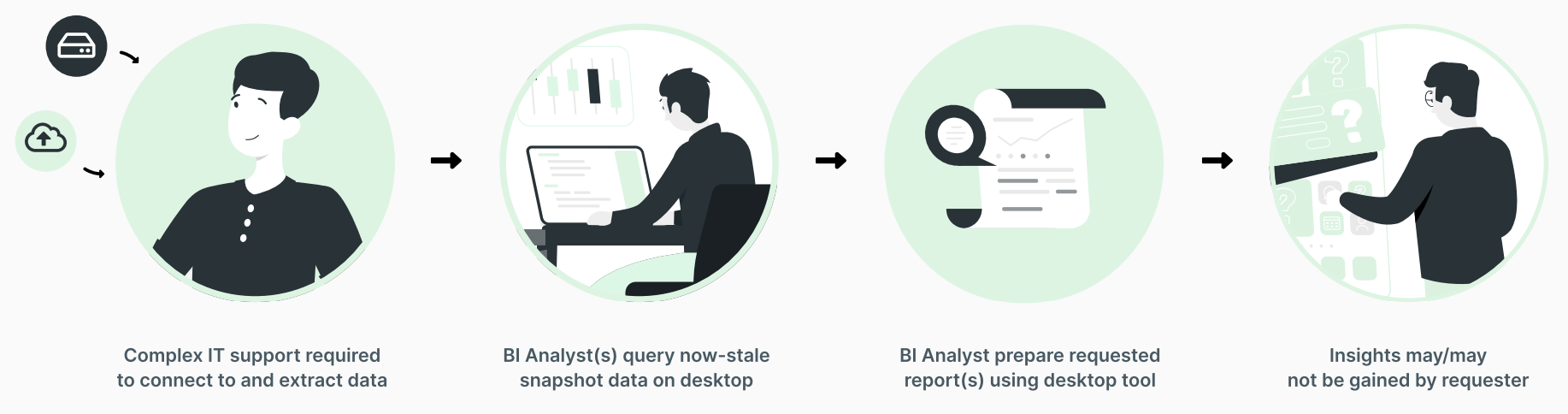
Chapter 3: Formulating a Data Strategy
Many private equity firms have data systems that don’t interact, even though they are relevant to each other. While organizations and managers need to look at a more holistic view of data, there can be tremendous inefficiencies involved with trying to bring individual systems together, showing a lack of capabilities of many tools on their own.
Effective use of data coupled with a technology solution that is specialized for private equity firms can help organizations significantly improve their use of time and resources, increasing efficiency and simplifying processes.
There are solutions available that unite data from disparate sources into one place, then model the information together to establish a single source of truth for all of the data across all of the systems. Even better, technology can connect to the data systems already in place, with no need to export or upload information, simplifying the process. This facilitates very powerful and very modern data capabilities in terms of analysis on a large scale. For example, solutions like Altvia Answers can do this work in the cloud in an automated fashion, eliminating the need to embark on manual, time-consuming, and error-prone processes. Most importantly, solutions like this provide the ability for business users to interact with their data across systems in very intuitive ways, on any device, eliminating the need for highly skilled, IT-centric re- sources that would historically have spent weeks at a time developing an analysis. A good solution should be right at the fingertips of business users and executives at any point, ready for them to ask their own questions and get their own answers in an engaging and intuitive way. Next are a few examples of data and technology solutions specific to the needs of private equity firms and managers.
Contact & Data Management
A contact and data management system (or “CRM”) is a technology for managing relationships and interactions with customers and prospects, helping companies stay connected to customers, streamline processes, and increase profitability.
This type of solution helps organizations focus on relationships with individuals — in the case of private equity firms, that would include relationship management of stakeholders, users, investors, prospects, deals, fundraising, and more. These solutions can help a firm through life cycles, from finding new customers and winning their business, to providing support and additional services.
Altvia’s contact and data management solution, AIM, combines the Salesforce platform with a proven process to harness the institutional knowledge of communications, LP portal and back-end systems specific to the private equity industry. AIM helps firms easily and successfully raise and deploy capital, ensure compliance, and deliver a trusted and transparent experience to stake- holders and investors.
Business Intelligence & Data Analytics Solutions
The ability to use multiple, separate databases, but join them in a way that enables users to leverage the value of each together while also maintaining their independence provides the best of both worlds: flexibility and customization plus full coverage. Additionally, enhanced reporting capabilities enable custom views and data capture to be viewed and exported. Pushing this data to a data room or LP portal enables the ability to easily and securely share specific data with stakeholders and investors.
Here’s an example of how a solution like this could help a firm: an organization has spent nearly two years assessing vendors in an attempt to find a solution that could deliver on their vision. They are looking for a solution that would allow them to provide a highly differentiated service that is unique and superior to their competition. After assessing dozens of vendors, they found a few that came very close to meeting their criteria, but there was always something missing from the bigger picture.
What they needed was a provider that really understood their vision and helped them to identify the best of breed, best in class solution to work across and connect their entire chain of systems. They needed a solution that al- lowed them to use the best contact and data management system, then put technology on top to connect to the rest of their systems and databases. An entirely connected system would provide that firm with a single place for business users to go in order to find and understand data across and throughout their organization.
Altvia’s analytics solution, Altvia Answers, is purpose-built to pull data from any disparate source into a single platform. Combining Altvia Answers with AIM, the contact and data management for private equity and ShareSecure, Altvia’s LP portal, ShareSecure, enables a powerful, integrated system with immense capabilities for firms and investors.
Data Warehousing & ETL Technology
In relation to the private equity marketplace, data is making substantially more appearances in industry studies, headlines, and even keynote topics at industry events. While data has long been a source of pain for firms, increasing competition and demands are driving an increased priority to have faster and simpler access to more data.
Along with an increase in the need for accessibility to growing and complex data sets, terminology that pri- vate equity firms need to be aware of to operate more effectively is growing as well. Buzzwords like data ware- housing and ETL are becoming more common, and it can be helpful for firms and managers to know what these processes are, and how they can help private equity firms differentiate and grow more efficiently.
Data Warehousing
On a high level, a data warehouse is designed to store and warehouse data to allow technology to provide lever- age. In the absence of data warehouses, data is stored in many different places and any number of different versions. Data warehousing technology is key to solving business challenges, and it helps to bridge the gap of what investors and customers don’t have access to. Data warehousing is the link that connects data while enabling stakeholders and customers to leverage the value of each independent database. This dramatically improves operational efficiency for firms.
ETL
Organizations need to acquire data, prepare it, analyze it, and consume it in order for it to provide value. ETL (extract, transform, load) is the process of extracting data from its native location, then transforming and organizing it properly. Once extracted and set into usable for- mats, it can be served up for analysis and consumption in forms such as simple queries, visualization, charting, tables, and more. Extracting, transforming, and loading data is a technology process, and organizations can eliminate human error risk while saving time and resources by allowing technology to do these processes.
Data Warehousing + ETL
Combining the two concepts of data warehousing and ETL allows data to be stored in a single place. In addition, there are no questions as to version control issues and by allowing technology to do the steps, your firm’s skills and resources can instead prepare for analysis.
Solutions like Altvia Answers take advantage of this combination, allowing business users get the information they need themselves, without swivel chair between programs or waiting to get data from other teams. Data is instantaneously ready to be analyzed and consumed. Altvia Answers makes technology do the work, then enables the end consumer (typically business users) to interact with the properly-prepared data out of the ETL process, and in a matter of clicks in seconds, filter down the criteria they’re looking for, ask questions that they have, and get the answers back immediately in real-time without human intervention, time or risk. Technology should be at the core of a solution in terms of how problems are solved, freeing up teams to focus on more valuable work including analysis and deals.
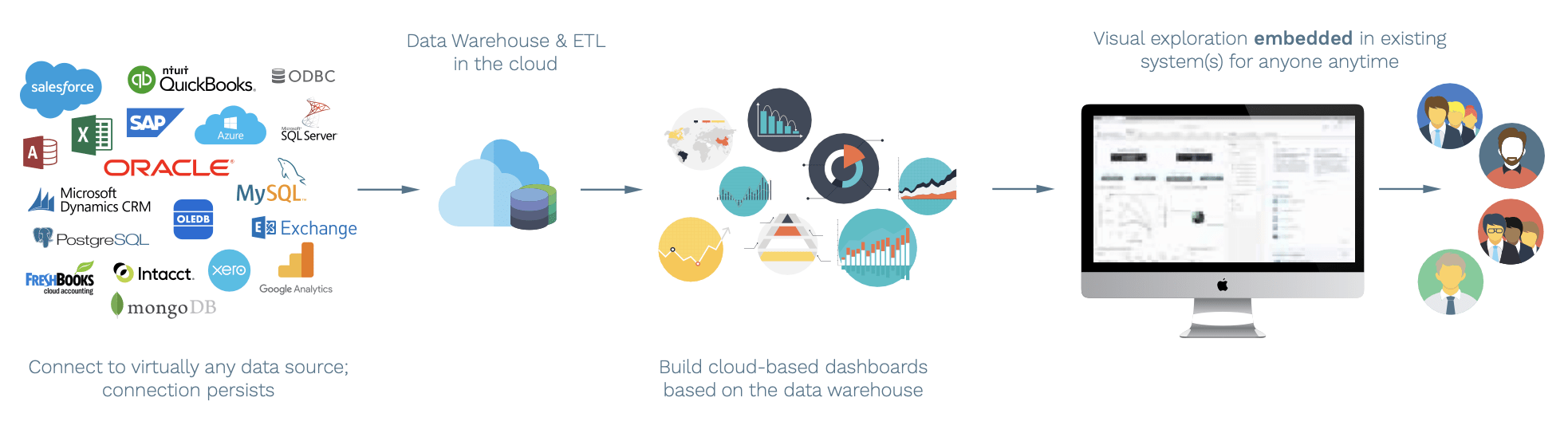
Chapter 4: Differentiation In The Marketplace
Beat The Competition With Enhanced Operational Efficiency
Data is growing in importance partially due to the fact that related challenges are becoming more persistent. Many organizations and managers are aware that data is the key to these issues, but may not know how to implement solutions that create leverage around data instead of hassle or struggle.
Data and technology solutions like Altvia Answers are designed to make working with data painless, easy, less error-prone, and independent of specific resources or wrong, miscalculated or outdated data. With Altvia Answers, a business user can get real-time data in three clicks, rather than three weeks, enabling firms to get time back to focus on finding new investment opportunities and prospects. Solutions like this are shaping the future of differentiation: the ability not only to answer the questions that people are asking, but to allow them to ask questions and answer them themselves as well. Not only can firms differentiate by optimizing operational competency, but can take it further by starting to enable customers, investors, or constituents to self-serve. That provides the level of differentiation private equity firms are chasing.
Leveraging Data & Technology As An Industry Leader
The value of data and technology lies not only in the ability to consume data across systems internally, but also to begin to allow clients themselves to access important, real-time data that is relevant to them.
With the right solution, a firm can go from sending a statement in the mail every so often to eliminating the need for stakeholders or customers to even pick up the phone to ask questions about data; they can simply access the information that’s relevant to them in real time, at any time, in an intuitive, efficient, interactive, and engaging way. This can have a snowball effect, helping to provide more efficient internal operations that are more organized and more systematic. This effect can trickle all the way through to providing new capabilities to firms’ investors, which in turn strengthens relationships and positions organizations as industry leaders.
How Firms Can Better Differentiate In The Marketplace
It’s not effective to throw technology at a problem and expect change to happen. The most successful organizations will consider change management and effective leadership in order to make significant strides forward. Also, while it may take longer to begin performing tasks in a more systematic manner, the initial, upfront investment of working in a smarter way is going to continue to pay dividends. This will help organizations better lever- age their human capital. Stepping back to get out of some of the mundane, manual operations that firms may still be using enables teams to work toward more fulfilling — and profitable — tasks such as raising capital, courting the right prospects, and sourcing better deals.
Thinking more strategically, as opposed to grinding away, is the mindset shift that will help firms to advance. It’s important for organizations to start with the right balance. Having an awareness of what technology or a solution can do helps to inform what might be possible, then raising awareness about those possibilities is what opens up an organization’s true potential. It’s all about bigger shifts that may take a little longer, as opposed to doing what’s quick and convenient right now, so that firms truly get the full value of the improved data and technology systems that are available.
Ready to Arm Your Firm With An Effective Data-Driven Strategy?
Fill out the form below to get started: